The integration of services powered by artificial intelligence (AI) is becoming a strategic lever for businesses. AI allows companies to transform how they interact with customers, optimize internal processes, and innovate in their service offerings. More specifically, it’s the industrialization of Large Language Models (LLM) that presents unprecedented opportunities for natural language processing (NLP). Gartner even states that AI ambitions should be a top priority for CIOs in the next 12 to 24 months (source: Gartner).
However, very few companies have the capacity to develop their own LLM, and the models available on the market (such as ChatGPT and others) do not have access to company data. Fortunately, there is an approach that makes LLMs accessible to a much wider audience!
Alexis de Saint Jean, Director of Innovation at Blueway, reveals this approach and the mechanisms that make AI industrialization possible, even for mid-sized companies.
Can you explain what an LLM is and how it relates to AI?
A Large Language Model (LLM) powers generative AIs like ChatGPT, Google Gemini, and Mistral (a French model!). It is based on deep neural network architectures, specifically the Transformer architecture, designed to process and generate natural language on a massive scale, incorporating an attention mechanism. These models are trained on terabytes of data (pre-training), allowing them to learn a wide range of contexts. Through unsupervised or semi-supervised learning on these large, general corpora, LLMs gain the ability to perform basic natural language processing (NLP) tasks such as text comprehension, content generation, or machine translation, by deducing relevant responses based on the provided context.
Their performance relies on the number of model parameters, often measured in billions, which capture complex relationships between elements of human language. Therefore, creating these LLMs requires substantial infrastructure. Training these models costs millions of dollars (for example, OpenAI’s LLM training cost $400 million over a single year, source: Fortune).
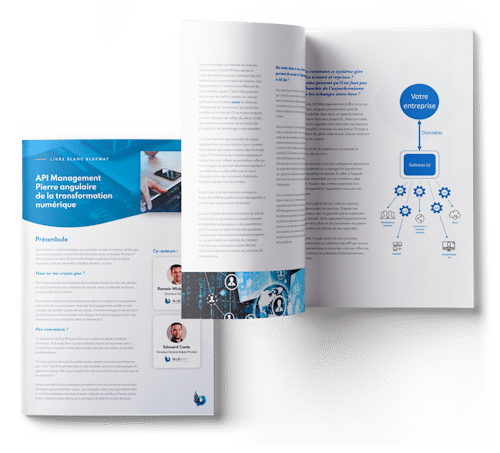
Download our White Paper on API Management
How Can SMEs and Mid-sized Companies Benefit from an LLM Since They Can’t Afford to Develop One?
The main challenge with these “generalist” LLMs is that they don’t have access to company-specific data that isn’t available on the web. As a result, in their current form, these models aren’t particularly useful to companies. The real issue is enabling these models to understand the “context” of the business — its vocabulary and information — without undergoing complex and expensive training processes, all while keeping control over the company’s data.
To address this challenge, there is a mechanism known as “RAG,” which stands for Retrieval Augmented Generation. RAG optimizes the output of an LLM using an internal company vector database. Essentially, this technique allows large language models to leverage additional data resources without the need for retraining. It’s a powerful way for businesses to take advantage of LLMs while maintaining data privacy and reducing costs.
How to Implement RAG Within the Company and Its Information System?
The Phoenix data platform can play a key role in implementing the mechanisms needed to power an internal RAG solution for the company. Practically speaking, a RAG system consists of two main components. The first is a vector database (DB), which, as the name suggests, stores vectors. In this context, these vectors represent documents specific to the organization, which are processed and segmented so they can be injected into this database. The first step involves data retrieval, processing, and writing.
Phoenix orchestrates and industrializes all these retrieval and injection mechanisms through its multiple connectors. By automating these tasks, Phoenix ensures that the internal RAG solution runs efficiently and can continuously update and manage company-specific data, making it accessible to the LLM without the need for extensive retraining. This integration allows the company’s information system to fully leverage AI while keeping data control and streamlining operations.
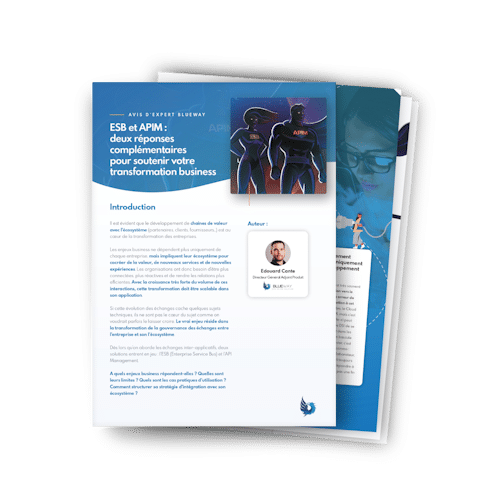
Download our expert view on the complementary use of ESB and APIM solutions
How Can One Interact with the LLM if It’s External to the Organization?
When a question is posed via the LLM, it is first transformed into a vector and then sent to the RAG system to search for the closest vectors within the vector database. This process is a form of semantic similarity search, which retrieves the most relevant documents to provide the context the LLM needs. This context then enables the LLM to construct its response and generate text accordingly.
In this way, interaction with an LLM and your organization’s own data becomes possible. To summarize, if the vector database is the first component of the RAG system, the second component is its ability to interface with the LLM. Phoenix supports the implementation of this RAG/LLM retrieval and query mechanism via APIs, allowing for end-to-end industrialization of the process so companies can fully benefit from AI.
Furthermore, Phoenix can expose this RAG to any LLM, even through AI marketplaces like OpenAI. OpenAI, for instance, provides access to “GPTs,” which are agents specialized in various fields, allowing businesses to leverage specialized AI solutions in a streamlined way.
Does This Mean Losing Control of Your Data?
Not at all! By using “GPTs” via a RAG system, you can harness the power of ChatGPT with your company’s data without ever exposing it, since the LLM doesn’t need to be trained on your data. Thanks to Blueway’s API Management platform, it’s possible to securely use the content from the vector database. The external LLM retrieves the necessary information to build its response through API calls, but the data itself is not sent to OpenAI or any other LLM provider.
These API calls are secured, traceable, and revocable through centralized tokens. Phoenix thus acts as a key facilitator in creating value-added applications using LLMs and AI, while ensuring data control and security throughout the process.
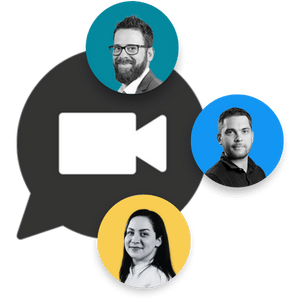
Want to discuss API Management with an expert ?