Data processing is frequently reduced to data cleansing and then integration, but doing so means an incomplete picture of the process is being used. To fully exploit data, the entire data lifecycle has to be considered. Carefully managing each stage in data processing, from collection to storage, and putting the right tools and methods in place at key waypoints, will improve the effectiveness, quality and therefore the usability of your data. The entire organisation, from functional units to the C-suite, will be able to gain greater value from correctly processed data.
What exactly is data processing ?
Data processing refers to the operations applied to data from the point it is collected onwards, so as to make it useable and maximise its value. This process has to be structured and run continuously so it is not overwhelmed by the sheer volume of data collected daily.
While data processing can include manual processing – for example a data scientist can in some instances take charge of the first phase of processing and produce documented analyses that then join the IS and its business applications – it also includes a number of automated stages. In general, data processing entails the following phases :
- Collection: data – structured or otherwise – is collected from all available sources (data lakes, data warehouses, Excel, business applications, IoT and so on).
- Pre-processing: the data is cleansed, duplicates removed and low quality data is discarded.
- Data import: the data is converted into a usable format and transported to the leading IS applications.
- Processing: automated solutions are used at this stage to structure and transform data for future use.
- Data exploitation: once available, the data can be queried and used (by the business, BI systems, functional processes, etc.) whether for strategic decision making or quite simply as part of the organisation’s various activities.
- Storage: data processing also includes the storage phase, which is important to keep data in compliance with the GDPR and archiving standards, depending on the context.
The profile of the data collected at the outset and its subsequent processing are both important quality factors: data will only provide added value if it is from a trustworthy source, and then properly structured during pre-processing, on the basis of the intended end use.
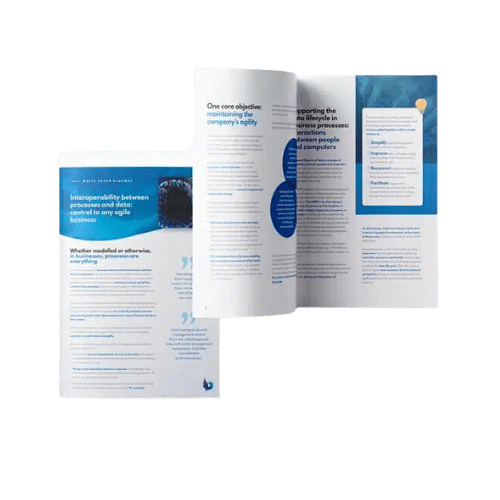
How process (BPM) and data (ESB) integration can create value for IT and business departments ?
How to ensure effective data processing ?
At the crucial integration stage, data processing brings into play solutions such as ETL (Extract Transform Load), EAI (Enterprise Application Integration) and the application bus or ESB.
But adding value to data does not depend solely on integration. Data transport, storage, the efforts made to structure it and improve its quality… each of these waypoints brings about changes to the data and therefore plays a major role in its usability.
Another essential factor in successful processing is taking all specific needs into account, including any industry-specific standards and formats, functional business requirements, and coherent data traffic management. Adapting processing to fit the business makes a real difference.
A number of criteria combine to meet the needs of each business:
- Automated supervised integration: a supervision console can be used to monitor traffic status, KPIs, ratification procedures, security, etc. and it provides visibility over data interchanges, processing and integration.
- Standards-compliant interchanges and smart routing: integration solutions must accelerate data exchange, and therefore immediately convey data to the right applications while ensuring persistence if necessary (synchronous/asynchronous). In operation, the solution also needs to convert messages into a standard and, if necessary industry-compliant, format.
- Uncomplicated data manipulation and enrichment: in addition to intelligent mediation, it must be possible to readily configure and fine-tune data processing, and to enrich messages by means of intermediary distribution circuits (such as a master data repository).
But successful data processing does not stop there, of course. The quality and usability of data are relevant issues throughout its lifecycle; at the moment a data repository is built, when orchestrating data traffic to fit with the sequence of business events, during context-specific data provision, and so on. 360° data processing is not only about solutions that add value to data, and the overall approach must also factor in the final use that will be made of that data.
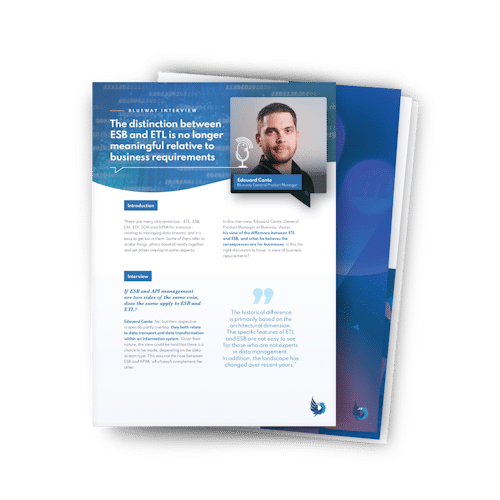
ESB vs ETL ? The distinction between is no longer relevant relative to today’s business requirements.
ESB, BPM and MDM are the tripod supporting the data lifecycle and effective data processing
The ESB (Enterprise Service Bus) forms the foundation of the Blueway solution for complete data processing (data manipulation, management and exposure, following an SOA model. The application bus does play a major role in the data interchange structuring stage, that role being to facilitate the circulation of data between functional business applications and to re-engineer the information system.
However, our approach at Blueway takes all the other aspects of data traffic management into account. It is only in this way that the value the data brings to the organisation can be maximised/
- MDM: upstream, MDM (Master Data Management) is the single master data repository that ensures the successful collection, quality control and enrichment of the data that is to circulate around the IS.
- BPM & APIM: Downstream, Business Process Management ensures that the right data is supplied at the right time, to fit with the work of business users. It also provides interfaces for the input of structured data, on the basis of the usage context. These can also be exposed to partners using our API Management solution.
Together, these dovetailing components integrated within the single Phoenix platform mean control and visibility can be kept over the entire data lifecycle. Phoenix facilitates implementation of an SOA architecture and adds real value to data, adapting processing to each organisation’s requirements, from data collection to strategic decision making.
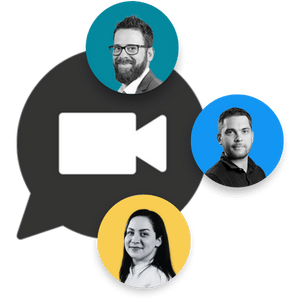
Want to discuss interoperability challenges with an expert?