Data quality assurance: obvious and vital work
All business processes are based on data nowadays, and the same applies to project analysis and management. Data-driven strategies are becoming the norm; data quality assurance is therefore necessarily a central topic.
However, crucial though the subject is, dealing with it remains on hold in many businesses, because the issues surrounding data quality are not always accurately quantified, and the organisation to be put in place, and deciding priorities, both give rise to many questions.
Data quality affects each link in the information chain. Pointless extra costs, wasted effort, poor decision-making… the consequences are important as regards:
- Processes and process execution: inaccurate processes worsen the quality of the end product or service, are less efficient, and give rise to errors that can be critical if the process is a sensitive one.
- Customer relationships: in discussions with after-sales or any other CRM-related interaction (e-commerce website, customer newsletters, etc.), data must be accurate if problems are to be solved and questions answered quickly, and the company’s image is to be maintained.
- Strategy choices: examining situations using poor data runs the immediate risk of taking unsuitable decisions or selecting inappropriate solutions.
While not a complete list, these three issues show the importance of data quality work.
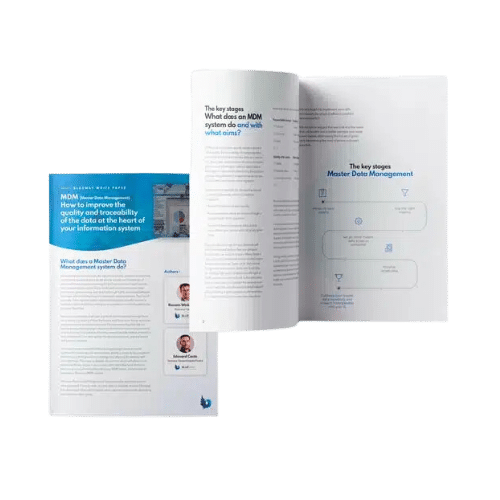
Master Data Management : data quality and traceability at the heart of your information system
What steps are involved in data quality assurance?
Ensuring data quality is not a “Big bang” exercise. You need to fully understand the organisation, and the work must be structured so as to cover every aspect and properly delimit the project scope.
Data audit and determining data usages
You have to know your data before you can work on its quality. It is important to audit your data in terms of data definitions, management, the processes using it, etc. and to produce a data map.
Data quality really depends a great deal on the uses to which the data will be put. The essential factors that will determine quality assurance must therefore be decided upon first. Such factors are likely to focus on the accuracy, completeness, relevance, age and the consistency of the data.
The objectives, requirements and expectations of business departments should govern choices around format, content and availability. It is crucial that data can be quickly used by all the applications concerned.
Alongside determining the future uses of data, a detailed audit of existing uses must be conducted. A statistical analysis of the data will reveal the current situation (irregularities, duplicates, values) and the relationships between all the data sets.
Setting the rules for data formulation and governance
The next step is deciding the rules, organisation and tools to be used. This will provide control over, and access to, data within the desired timeframe, following the data validation route chosen.
The following points are particularly useful when putting data governance in place:
- The content of metadata: describing both the type of data concerned and the processing the data undergoes. Semantic metadata should therefore be managed comprehensively and in detail to ensure all users can easily find the right data sets to suit their requirements.
- Data search and unification tools: the data dictionary which lists and categorises all the data in the business, the data glossary which gives semantic explanations and contextualises data, and the data catalogue to connect the dictionary and glossary, all help to align the perspectives held about the data by the IT department and functional business areas respectively.
Unified data better meets the functional requirements of the business, and is easier to share. - Roles must also be defined to check on data and data enrichment. Positions such as the Chief Data Officer, or the more operational Data Steward, or the Data Quality Manager, will be directly responsible for data quality and the preparation of data for use by the business quickly. Such roles also include the preparation of data distribution in a highly demanding marketplace (imposing a need to manage the data lifecycle, traceability and regulatory compliance). More broadly, they also help to promote a “data culture” in the organisation.
Beyond these operational points, the business should take a step back to gain some perspective on its data strategy and how its governance is organised. This can include setting up a data governance body, and a cultural induction of staff on the issues surrounding data, or “data literacy”. Any such measures should always be adopted company-wide and with senior management’s active backing.
Once the scope has been settled, the quality assurance work itself can begin.
Before implementing a solution, it is important to design a trajectory and the key stages. Both technical and functional views need to be combined when considering the options here; formatting data for functional needs will only be useful if data streams are under control and solutions integrated within the IS, and vice versa.
Solution selection and implementation
Data quality assurance work is multi-faceted. Addressing the three dimensions, i.e. data, streams and processes, is the best way to comprehensively cover all the angles when considering data centralisation and harmonisation. It is crucial that full data integrity is maintained, from the time it is collected to the point of use in the business.
Combining MDM, ESB and BPM solutions means data is examined from every viewpoint, reconciling the technical aspects and business requirements.
MDM
(Master Data Management) compiles a single, high-quality data record, with duplicates removed by following customisable functional rules, offering traceability and QA, and data monitoring throughout its lifecycle. The module automatically generates data acquisition and exposure web services, harmonising data that will be shared across the entire information system.
ESB
The application bus standardises data traffic, thereby helping to maintain the single view of that data that can be used by all applications during its entire lifecycle. MDM and ESB interact both ways, with the master data enriched by business applications themselves.
The data transmitted to applications in the IS is secure (encrypted, compressed, and subject to validation procedures) and the use of semi-connectors helps minimise data transformations.
BPM
Business Process Management sustains data throughout its lifecycle and makes it available to benefit users. The various functional areas enrich data and increase its value through their business processes. This approach ensures that processes are truly embedded in the IS, with high interoperability between processes and data.
Regardless of the solution, or combination of solutions, chosen, it is important to proceed step by step. As we explained, a “big bang” approach is not suitable to work on ensuring data quality. The trajectory will be defined on the basis of the priority data scopes, to make progress step by step and consolidate as the project goes along. For each, data is to be thoroughly prepared (collected, duplicates removed, missing items added) and connectivity to applications checked as implementation progresses to deliver benefits quickly. Implementing ESB, MDM and BPM modules that communicate poorly is not the aim!
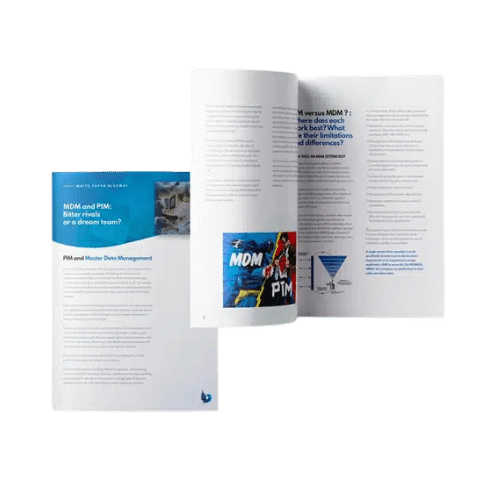
MDM versus PIM: bitter rivals or a dream team ?
Real-time fixes and continuous improvement of data quality
In addition, data quality assurance does not stop once the solution has been implemented. Organisational changes and changes to applications in the business must be tracked as and when they occur.
This means splitting human activities from the technical side. People are an integral part of the chain via the user interface, offering the possibility to enrich, correct, confirm, etc. data.
Measurement, and reminder and warning systems should make it possible to identify and fix the most significant data quality problems in real time. An incident-handling system will make it easier to trace the source of errors. Reports, a dashboard and task allocation enable processes to be radically improved, and data entry errors and other causes of irregularities to be corrected. A management console of this kind must also include supervision of data streams and processing, as well as data quality.
It should therefore be possible to flag data quality problems to the relevant business area as soon as they arise, as well as regularly producing reports and KPIs. This makes it possible to introduce best practice in data entry and to instil data quality assurance into the business culture.
Automation, meanwhile, should help to ensure that the rules determined at the outset are still followed. The solutions chosen should mean that data is always checked and confirmed before it is added to the master data records. Greater quality in a shorter time!
At Blueway, we firmly believe that data, data streams and processes are interdependent and that each of them contributes to data quality. Data governance and the solutions to be implemented must therefore incorporate all of these aspects. And that is the purpose of the Blueway platform.
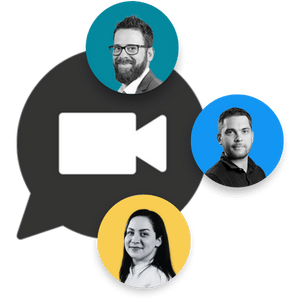
Want to discuss your data management challenges with an expert?